Content
Recent Posts
Goldman Sachs: Is AI Failing to Deliver?
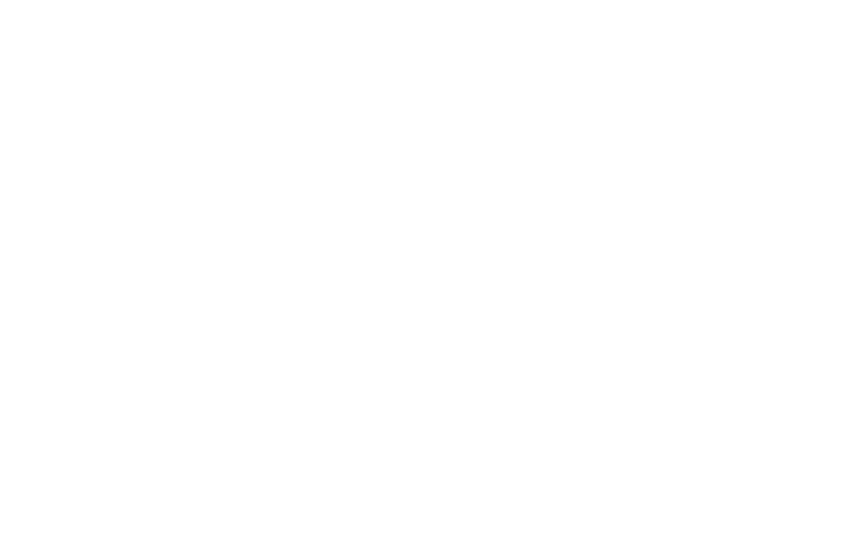
July 17, 2024
Artificial Intelligence (AI) has been heralded as a transformative force across various sectors, promising unprecedented efficiency, insights, and automation. However, Goldman Sachs' recent report presents a more skeptical view, questioning whether the current enthusiasm for AI is justified given the associated costs and challenges.
Content
The High Financial Burden
Goldman Sachs highlights that the financial burden of implementing AI systems is substantial. These costs are not confined to the initial investment in AI technologies but extend to ongoing expenses such as:
- Data management: High-quality data is the lifeblood of AI, necessitating significant investment in data collection, cleaning, and storage
- Infrastructure: Advanced hardware and software infrastructures are required to support AI operations, leading to continuous capital expenditure
- Talent acquisition: Skilled AI professionals are in high demand, driving up the costs of hiring and retaining talent
Uncertain & Variable Returns
Despite significant investments, the returns on AI can be uncertain and highly variable. The report underscores that many businesses struggle to achieve a satisfactory return on investment (ROI) from their AI initiatives due to several factors. The effectiveness of AI can vary significantly across different industries; while some sectors, like finance and healthcare, may see immediate benefits, others may experience delayed or marginal gains. Additionally, successfully integrating AI into existing workflows is complex and often requires substantial time and resources, with missteps in implementation leading to costly setbacks.
Reliability & Bias Concerns
Goldman Sachs also raises concerns about the reliability of AI systems. Despite technological advancements, AI models are not infallible and can produce erroneous results due to data bias and model errors. AI systems trained on biased data can perpetuate and even exacerbate existing biases, leading to unfair and unreliable outcomes. Moreover, complex AI models, particularly those based on deep learning, can make mistakes that are difficult to diagnose and correct, especially in critical applications like medical diagnostics or autonomous driving.
Misalignment of Expectations
The report suggests a significant misalignment between the hype surrounding AI and its actual performance. Overzealous expectations fueled by media and industry proponents often clash with the practical realities of AI deployment. Many businesses use AI with unrealistic expectations about its capabilities and immediate benefits. Data quality, integration, and scalability challenges are often underestimated, leading to disillusionment and frustration.
Strategic Recommendations
Goldman Sachs' report concludes with strategic recommendations for businesses considering AI investments:
- Realistic goal setting: Businesses should set realistic, measurable goals for their AI initiatives, aligning expectations with the current state of technology
- Incremental implementation: Rather than attempting sweeping transformations, companies should adopt an incremental approach, allowing time for adjustment and learning
- Continuous evaluation: Ongoing evaluation and adjustment of AI strategies are crucial to ensure that investments yield the desired outcomes and adapt to evolving technological landscapes
What This Report Does Not Consider
While Goldman Sachs' report raises valid concerns, it overlooks instances where AI has already benefited numerous companies by executing time-consuming tasks efficiently. When given specific, well-defined problems, AI can produce quick and efficient results, building confidence and expertise in the technology. For instance, automating routine administrative tasks or enhancing customer support with AI-driven chatbots has significantly improved efficiency and customer satisfaction. These smaller-scale projects typically require less investment and can be scaled gradually, allowing companies to utilize AI benefits while focusing on essential tasks for business growth. These small projects have already provided opportunities for companies to build internal knowledge and capability, which is crucial for more ambitious AI initiatives. Each small success builds a track record that can justify further investment and innovation in AI.
Conclusion
Goldman Sachs' report presents a skeptical view of AI, highlighting substantial financial burdens, uncertain returns, and reliability issues. It also points out a significant misalignment between high expectations and practical realities, suggesting businesses set realistic goals, adopt incremental approaches, and continuously evaluate their AI strategies. However, the report overlooks AI's successful applications in automating tasks and improving efficiency in smaller-scale projects. These successes build confidence and internal expertise and serve as the base for more ambitious AI initiatives.